Data Quality
When talking about data quality, we think of the accuracy, completeness, consistency, and reliability of data. It directly impacts the effectiveness and efficiency of business operations and overall organizational performance.
What Is Data Quality
Data quality is about how well data meets certain standards. These standards include accuracy, validity, completeness, and consistency. Think of it as a way to check if the information your company uses is reliable. Poor data quality can cost businesses a lot, about USD 12.9 million each year. This shows how important it is for success. If your data is wrong, it can mess up important decisions.
Keeping data consistent is also vital. It means the data doesn't contradict itself. If it does, it can lead to bad decisions. Companies that focus on data quality do better and make customers happier. Good data quality is even more important in areas like hybrid cloud, IoT, and edge computing. These areas deal with big data, which can be complex.
Why Is Data Quality Important
CEOs worry about the data they use for decisions, showing its importance. Bad data can cost the U.S. economy $3.1 trillion a year, as IBM found.
It can cause mistakes like lost sales and shipping errors. Good data lets your team do their jobs better. This boosts growth and customer satisfaction.
In finance, quality data is a must for following rules and avoiding big fines. It helps you make smart decisions and understand your customers better. This improves your marketing efforts.
Investing in quality data makes your marketing campaigns more effective. Most senior executives see the value in using AI for better data management.
Components of Data Quality
Understanding the key parts of data quality helps you make better decisions. Each part is important for the quality and use of your data.
Accuracy
Data accuracy means the information is correct. This contributes to trustworthy reports and smart decisions. Accurate data avoids costly mistakes and gives you reliable info.
Completeness
Completeness checks if all needed data is there. This includes main data and extra details. For customer data, it means having the right info for good engagement.
Consistency
Consistency means data is the same everywhere. Different data can cause problems. Analytics should get the most from your data.
Reliability
Data reliability means it's dependable over time. It helps you trust your data and report accurately. Reliable data builds trust and keeps operations smooth.
Timeliness
Timeliness is about data being current. Having fresh data helps in making quick and smart decisions.
Validity
Validity checks if data follows business rules. For example, ZIP codes must be correct. It's a big part of data quality.
Uniqueness
Uniqueness stops duplicate records. It keeps your data clean and reliable, and contributes to data integrity.
Relevance
Relevance asks if data is useful. Useless data wastes time and resources. It's important to match data collection with business goals.
Accessibility
Accessibility means data is easy to get. Easy access helps your business move faster. It lets people use reliable info quickly.
Security and Privacy
Security and privacy protect data from misuse. Following rules keeps sensitive info safe. It protects your business and customer trust.
Data Quality vs. Data Integrity vs. Data Profiling
Data quality checks if data is right, complete, and up-to-date. Data integrity means the data must be whole, correct, consistent, and right for the situation.
Data integrity has six main parts: data integration, data observability, data quality, data governance, location intelligence, and data enrichment. Companies that focus on data quality can get better results from their data use. Using tools like the Precisely Data Integrity Suite can make your data more accurate and relevant.
Keeping data quality up can be tough, especially as businesses change. Companies deal with lots of different data, making data profiling harder. This step checks data for oddities and makes sure it meets quality standards.
- Fixing data quality issues early leads to better data integrity.
- A flexible approach lets companies customize their data integrity plans.
- Good data integrity is vital for business success and better results.
Not having enough resources can make it hard for companies to manage data quality and profiling well. It's important to invest in good tools and people to handle the complex differences between data concepts well.
Benefits of Good Data Quality
High data quality helps any organization aiming for success. It leads to big improvements in many areas. This boosts overall performance and helps in making smart decisions.
Informed Decision-Making
Good data means better decision-making. When you trust your data, you make more impactful business choices.
Increased Efficiency
Clean data makes operations smoother. It saves time and reduces errors. This lets your team focus on goals and progress.
Enhanced Customer Satisfaction
Quality data leads to better customer service. Accurate data helps personalize services. This builds loyalty and boosts sales.
Cost Reduction
Good data saves money. It cuts down on costs for fixing errors and avoiding fines. This improves your financial health.
Regulatory Compliance
Many industries must follow strict data rules. Quality data makes it easy to meet these standards. This avoids penalties.
Better Risk Management
Quality data helps spot risks early. Reliable insights help create strategies to manage risks.
Improved Collaboration
Consistent data improves teamwork. It leads to better communication. This boosts cross-departmental efforts and overall performance.
Competitive Advantage
Organizations focusing on data quality stand out. They use quality data for smart decisions. This gives them an edge over competitors.
Data Governance
Quality data supports strong data governance. It ensures data is accurate and relevant. This leads to better data management.
Long-term Sustainability
Investing in data quality is for the long haul. It supports growth, adaptability, and resilience.
Assessing Data Quality
Assessing data quality needs a structured approach. Focus on different criteria and use specific tools to improve your data's clarity and reliability. Here are some key steps to follow:
Define Data Quality Criteria
Set clear metrics for what quality means to your organization. This could be accuracy levels, conformity, and data uniqueness. For example, aiming for 85% accuracy while striving for 100% guides your efforts.
Data Profiling
Use data profiling tools to deeply examine your datasets. Good profiling helps spot issues like duplicates or missing data. This lets you focus on fixing these problems.
Data Quality Metrics
Use specific metrics to keep track of data quality over time. This might include data to error ratios and empty value counts. Regularly checking these metrics shows how your data is improving.
Automated Data Quality Tools
Automated tools make assessing data quality easier. They help check data regularly and fix problems early. This leaves room for ongoing data quality improvement.
Conduct Data Audits
Regular audits help in finding and fixing quality issues. When you systematically review your data, you ensure it meets quality standards. This keeps your data reliable.
Stakeholder Involvement
It's important to involve stakeholders in data quality efforts. Their input can provide valuable insights. This ensures a thorough evaluation that aligns with your organization's goals.
Implement Data Quality Frameworks
Using established frameworks standardizes your data quality assessments. This makes your practices consistent and supports effective data management.
Continuous Monitoring and Improvement
Always evaluate and improve your data quality practices. Regular reviews help refine your methods. This keeps your data up-to-date and reliable.
Documentation and Reporting
Keep detailed records of your assessments and changes. Good documentation helps track progress and informs data quality improvement decisions.
Training and Awareness
Teach your team about data quality standards and best practices. Training raises awareness and promotes a culture that values data quality.
Addressing Data Quality Issues
Managing data quality issues contributes to business success. First, find and fix errors in your data. Analysts spot and fix these problems.
Identify Data Quality Issues
Finding data problems early helps avoid big risks. Common issues include:
- Duplicate records from mistakes or system bugs.
- Wrong data from typos or old info.
- Missing data that hinders analysis.
- Varied data formats from different sources.
Categorize Issues
After spotting issues, sort them by impact. This makes fixing them easier and faster.
Root Cause Analysis
Understanding why data problems happen is the foundation. This way, you can prevent them from happening again.
Develop a Data Quality Improvement Plan
A good plan outlines steps, resources, and timelines for better data. It should match your company's goals and change as needed.
Implement Data Governance Policies
Strong data policies keep data clean and safe. They ensure quality and protect privacy.
Utilize Data Quality Tools
Use special tools to find and fix data problems. Tools like Secoda help keep data accurate. Automation makes fixing issues faster.
Train Staff
Teach your team about data quality. Workshops help them spot and solve problems.
Monitor and Measure Progress
Track data quality to see if your efforts work. Regular checks show what's working and what's not.
Engage Stakeholders
Get everyone involved in improving data quality. Their input helps everyone work together towards goals.
Continuous Improvement
Data quality work is ongoing. Always look to improve and adapt to new trends. This keeps your data quality high over time.
Data Quality Challenges
Companies face many data quality challenges. These can affect how they make decisions and work efficiently. For example, Gartner says bad data can cost businesses about $12.9 million a year. This happens when data is wrong or old, leading to wrong conclusions.
Inaccurate Data
Bad data can mess up plans and insights. It often comes from mistakes when entering data or when information gets outdated. For example, old customer addresses can cause problems with communication.
Incomplete Data
Missing data is another big problem. It can lead to unfair results. Companies need to clean and check their data well to make sure it's complete and right.
Inconsistent Data
When data doesn't match up, it can cause confusion. Make sure data is the same everywhere. This way, companies can trust their data and make good comparisons.
Duplicate Records
Duplicate data can slow things down and make decisions harder. Special software can find and fix these problems. This helps avoid having the same data twice.
Data Silos
Data silos hide important information. Companies should work to share data better. This helps everyone see the big picture.
Lack of Standardization
Not having the same way of managing data can cause problems. It's important to have clear rules for data. This makes things run smoother and improves data quality.
Changing Data Sources
When data sources change, it can mess with the quality of data. Companies need to check the new sources carefully. This helps keep data reliable.
Limited Data Governance
Not having good data rules can make things worse. It's important to have clear rules and someone to watch over data. This helps manage data well.
Regulatory Compliance
Following rules for data can be tough. Companies must keep up with these rules while keeping data good. This is a big challenge.
Evolving Business Needs
As businesses grow, their data needs change too. Companies should check their data plans often. This makes sure they match current goals.
Insufficient Resources
Not having enough to work with data can slow things down. Companies should invest in tools and people to make data better.
Resistance to Change
Some people might not want to change how data is handled. It's important to get everyone on board. Training can help make everyone understand the need for better data.
Complex Data Structures
Complicated data can be hard to manage. Making data simpler can help keep it accurate. This makes it easier to find the right information.
Lack of Awareness
Not knowing about data problems can stop companies from fixing them. Teach people about data quality. This helps create a culture that values accurate data.
Data Lifecycle Management
Manage data from start to finish. Companies need to have plans for every step of data's life. This keeps data reliable and useful.
Improve Your Data Quality with Kohezion
Poor data quality costs businesses about $3.1 trillion each year. Kohezion is a top-notch data quality improvement software. It makes managing data easier and more effective for organizations.
With Kohezion, you can create databases that fit your specific needs. This leads to more accurate, complete, and consistent data. It's a game-changer for your data collection and management.
Kohezion helps your business keep important information in one place. It streamlines processes and supports Master Data Management (MDM) strategies. These strategies help make quicker, smarter decisions that boost your business.
Good customer data management can also improve marketing and customer satisfaction. This drives your organization towards success.
Good data governance leads to clear and secure data use. It's important to train your staff on MDM practices and check data management performance. Only 15% of organizations have a solid data quality management plan.
With Kohezion, you can join the successful businesses that focus on data quality. This leads to better regulatory compliance and improved performance.
Frequently Asked Questions
Yes, relying on third-party data can introduce issues such as inaccuracies or inconsistencies, especially if the data sources are not vetted or regularly updated. It’s important to validate third-party data before integrating it with internal datasets.
Structured data, like databases, follows a clear format, making it easier to assess for quality. Unstructured data, like text or multimedia, lacks a predefined structure, making it harder to evaluate for completeness, accuracy, or consistency.
Real-time data quality monitoring systems continuously assess data as it’s collected, checking for accuracy, consistency, and completeness. Alerts are triggered if data quality falls below predefined thresholds, allowing for immediate action.
Data lineage tracks the flow of data through its lifecycle, from creation to transformation and usage. By understanding the path data takes, organizations can trace errors back to their source and improve data quality.
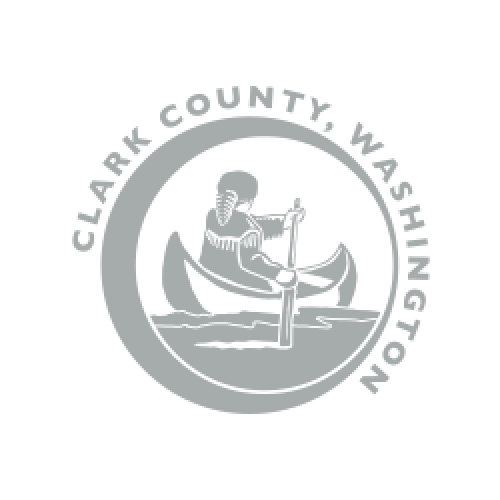
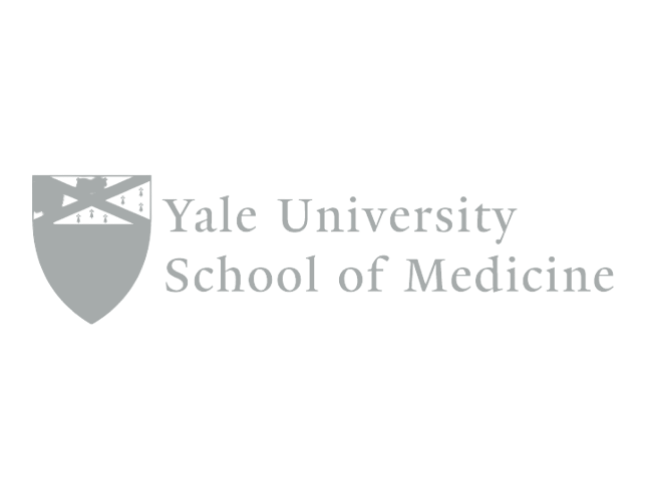
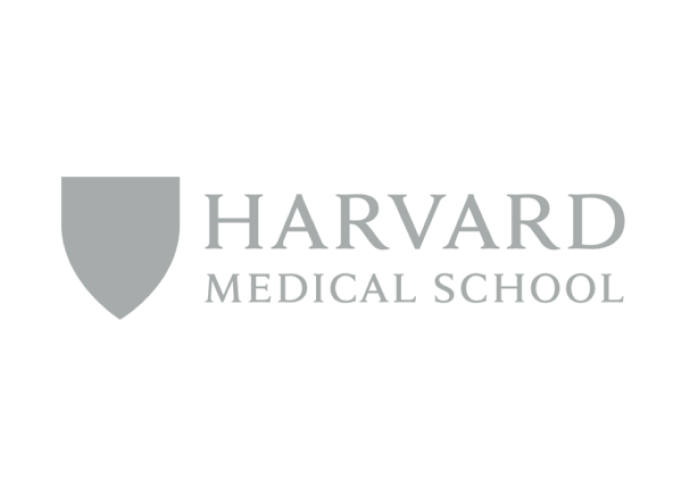
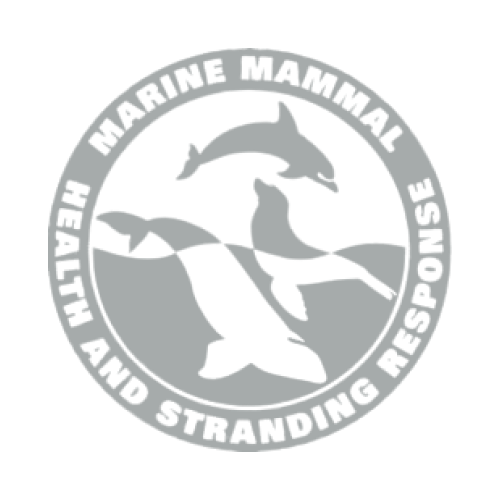
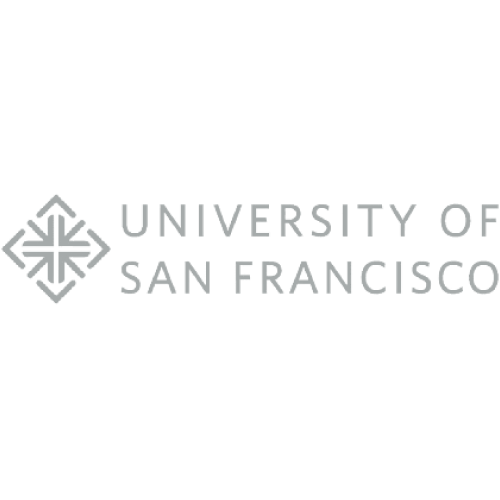
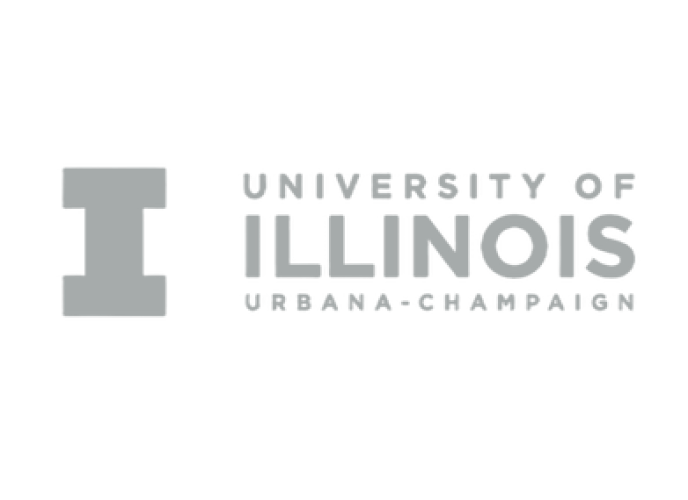